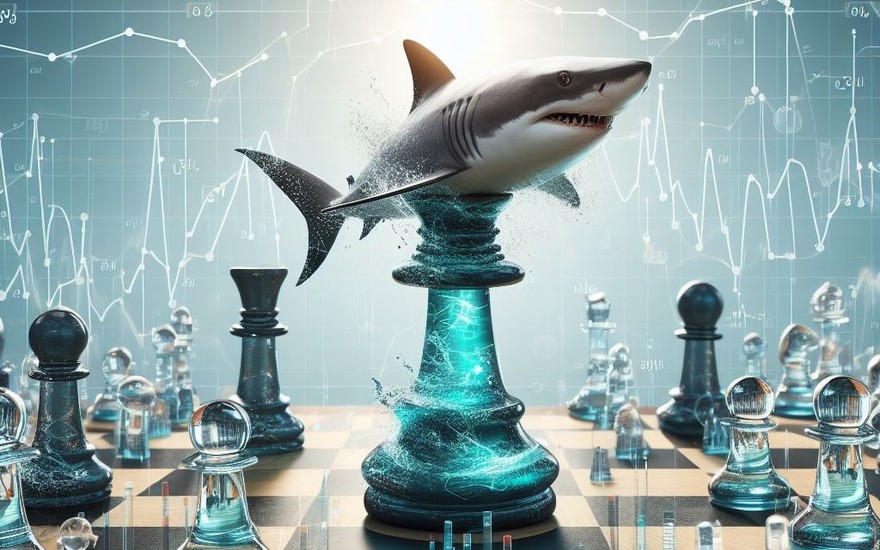
The xG of Chess: Shark Points
A metric to quantify missed chancesQuantifying chances in Football
Football (soccer) is a low-scoring game, with a large part of randomness. Counting the amount and quality of chances helps describe the balance of the game. The so-called ‘expected goal’ or xG are now ubiquitous. If a striker has a chance worth 0.2 xG, we expect that on average, he will score a goal one in five times. The model can be fairly complex and depends on the position on the pitch, the position of the defenders, the height of the ball, the position of the goalkeeper, etc. The models are often proprietary and created by large analytics companies and constantly evolved to include numerous factors.
Not one single metric is the be-all and end-all. Metrics are there to help and supplement the human analysis and understanding of the narrative. For example, the recent draw 0-0 between West Ham and Brighton was West Ham 0.82 - 2.66 xG Brighton. The xG allows us to see that clearly Brighton created several quality chances and could have deservedly won the game.
My friends of the Chess Pit Podcast and in particular football guru Jon McKenzie nudged me into developing a similar metric for chess. What if a game ends in a draw 0.5-0.5 but one player had a winning position?
Metrics are not a replacement for an actual understanding of the game, and the goal is to supplement the human understanding, not replace it.
Metrics are useful because they are objective and scale well: we can create averages over many games, while it’s difficult to remember details of many narratives and aggregate these in our heads.
This being said, let me introduce the xG of chess: shark points
Shark Points
“How to play queen to d6 in one second? It’s so undisciplined. You have to smell the blood in the water. Be a shark!” Magnus Carlsen
Shark points are based on the idea: how much would players score if they were perfect sharks?
If someone had a great advantage on the board but didn’t convert, he should score high shark points for this game
Let’s consider the game Ding-Rapport from the 2022 candidates
Ding had a win in the position as Qxd8 Nxc1? is met by Qg5!! Ding played Qxe2 and the game eventually ended in a draw.
Let’s compute the Shark point value for this game. The algorithm is the following:
The evaluation measured in centipawns alone is not very useful, because the meaning of a 50 centipawns change depends on the current eval value: it’s significant when the eval is zero and insignificant if the eval is 500. We apply a nonlinear transform to go from eval to expected score:
We could get very fancy and use a curve calibrated to the level of the players that we are analysing, but I’m using something very simple to start with.
Let’s look at the game Ding-Rapport from the candidates again:
So at most, Ding had a 0.92 expected score, which will be his shark points for the game.
Black never had the advantage so at best the eval was 0, so he scored 0.5 shark points. If the eval had swung in black’s favour, he would have had a higher shark score.
Drawback
Of course, the engine doesn’t take into account how easy the position was to play. This is a major drawback of the metric, but it’s okay because we should never rely on a single metric for everything.
Analysis of the Candidates Tournament 2022
For a single game, it will always be more interesting for a human player to have a look at the key moments. But over a whole tournament, metrics can really help understanding which player consistently wasted chances (low points score and high shark points score) and which player was a good shark.
For the candidates 2022, Nepo won convincingly with 9.5 points, second was Ding with 8.0. We all know the story: Magnus did not defend his title and Ding beat Nepo in the World Championship match.
What does the shark points table tell us? Ding had several missed opportunities like the one missed against Rapport, and he tops the shark points table with 10.36 points ahead of Nepo. We can say he’s a worthy second place in this tournament.
We could get into more complex analyses to see who is a good defender (e.g. saved the most positions) etc.
I hope the chess world embraces more analytics to improve and augment the commentary and the understanding of tournament dynamics. And remember:
Be a shark
Follow me on lichess and subscribe to my substack
More blog posts by BenjiPortheault
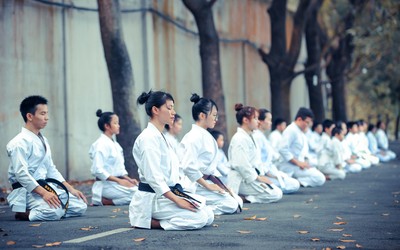
Discipline
An operating system to help you align actions and intent: the ICED framework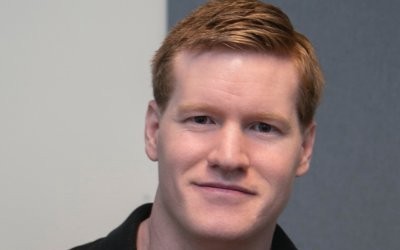
Guest Interview: Casey Thomas, Esports Performance Nutrition Specialist
How to think about nutrition and leverage it for chess performance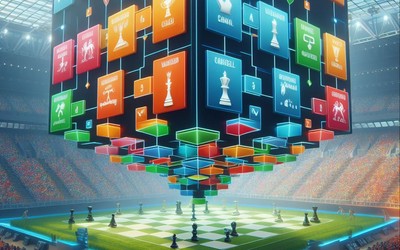
Tournament Formats in Professional Chess
Getting the conversation started on the drawbacks of ubiquitous formats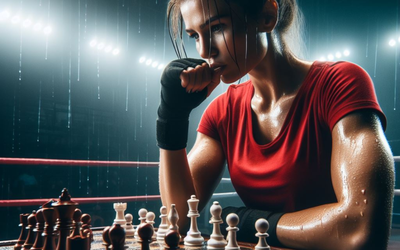